The 3rd Dutch Clinical NLP workshop is organized by a team from Utrecht University’s Natural Language and Text Processing (NLTP) group, Social and Affective Computing group, University Medical Center Utrecht’s Data Science and Biostatistics group, and VeiligheidNL.
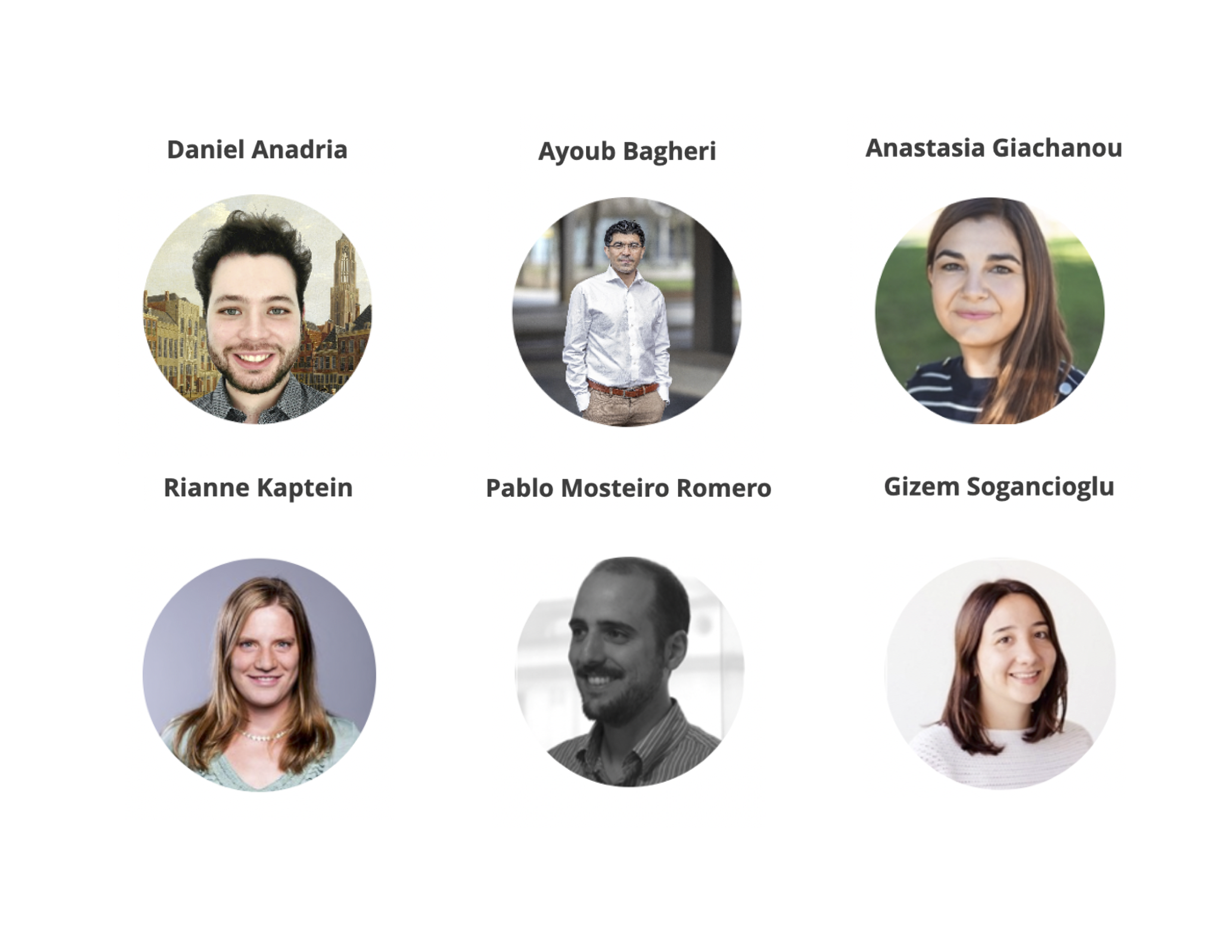
and Kiki Houwers who among other things has helped design this website.
The NLTP group works together on research projects to understand, interpret and model natural language. In addition, the group develops a wide range of open educational materials on text mining and NLP, as well as many tools for the community to use.
VeiligheidNL is the centre of expertise for injury prevention and has been working for 40 years to make the lives of millions of people safer by promoting safe behavior in a safe environment. The centre aims to achieve this through monitoring, expertise promotion, educating, and advising partners and professionals.
Some of our recent publications on the application of NLP in medical contexts include:
van Buchem, M. M., ‘t Hart, H., Mosteiro Romero, P., Kant, I. M. J., & Bauer, M. P. (2023). Diagnosis Classification in the Emergency Room Using Natural Language Processing. In Caring is Sharing – Exploiting the Value in Data for Health and Innovation (pp. 815 – 816). (Studies in Health Technology and Informatics; Vol. 302). IOS Press. https://doi.org/10.3233/SHTI230273
Sogancioglu, Gizem, Heysem Kaya, and Albert Ali Salah. “The effects of gender bias in word embeddings on patient phenotyping in the mental health domain.” 2023 11th International Conference on Affective Computing and Intelligent Interaction (ACII). IEEE, 2023. https://ieeexplore.ieee.org/abstract/document/10388203
Borger, T., Mosteiro Romero, P., Kaya, H., Rijcken, E., Salah, A., Scheepers, F., & Spruit, M.(2022). Federated learning for violence incident prediction in a simulated cross-institutional psychiatric setting. Expert Systems with Applications, 199, 1-9. Article 116720. https://doi.org/10.1016/j.eswa.2022.116720
Rijcken, E., Kaymak, U., Scheepers, F. E., Mosteiro Romero, P., Zervanou, K., & Spruit, M. (2022). Topic Modeling for Interpretable Text Classification From EHRs. Frontiers in Big Data, 5, 1-11. Article 846930. https://doi.org/10.3389/fdata.2022.846930
Rijcken, E., Zervanou, K., Spruit, M., Mosteiro Romero, P., Scheepers, F. E., & Kaymak, U. (2022). Exploring Embedding Spaces for more Coherent Topic Modeling in Electronic Health Records. In IEEE International Conference on Systems, Man, and Cybernetics (pp. 2669-2674). IEEE. https://doi.org/10.1109/SMC53654.2022.9945594
Teijema, J., Hofstee, L., Brouwer, M., de Bruin, J., Ferdinands, G., de Boer, J., Siso, P. V., van den Brand, S., Bockting, C., van de Schoot, R., & others. (2022). Active learning-based Systematic reviewing using switching classification models: The case of the onset, maintenance, and relapse of depressive disorders.
Van Steijn, Floris, Gizem Sogancioglu, and Heysem Kaya. “Text-based interpretable depression severity modeling via symptom predictions.” Proceedings of the 2022 International Conference on Multimodal Interaction. 2022.https://dl.acm.org/doi/abs/10.1145/3536221.3556579
Mosteiro Romero, P. J., Rijcken, E., Zervanou, K., Kaymak, U., Scheepers, F., & Spruit, M. (2020). Making sense of violence risk predictions using clinical notes. In Z. Huang, S. Siuly, H. Wang, R. Zhou, & Y. Zhang (Eds.), Health Information Science: 9th International Conference, HIS 2020, Amsterdam, The Netherlands, October 20–23, 2020, Proceedings (pp. 3-14). (Lecture Notes in Computer Science; Vol. 12435). Springer Cham. https://doi.org/10.1007/978-3-030-61951-0_1